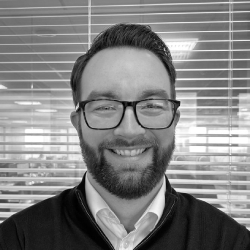
Mark Hughes, a commercial lawyer at O’Connors
Posted by Mark Hughes, a commercial lawyer at Legal Futures Associate O’Connors
The legal industry has traditionally struggled to keep up with technology. With artificial intelligence (AI) evolving quicker than any other technology, we need to be mindful of its limitations and walk before we run with these new innovations.
Almost every day there is a new headline relating to the development and growth of AI and how it promises to impact or improve on many tasks in daily life. With the ability of generative AI tools to create authoritative sounding responses to any query (or ‘prompt’) before your very eyes, it seems like almost anything is possible. At first glance anyway.
The reality of the situation is quite different. The current messaging from experts in the legal field is that AI will not be replacing lawyers any time soon. In fact, the application of AI may require even greater use of lawyers.
To better understand the limitations of AI, we need to understand the origins of it. After all, the concept has been around for years.
How does it work?
AI is the collation of information and a system’s use of rules to shape or extract an outcome from that information. The recent headlines are largely driven by the more recent and accessible iteration of AI, which is generative AI or ‘GenAI’.
These are formed on large language models that have been designed to apply many complex rules around ‘reading’ and ‘drafting’ language – most prevalently the English language – and then trying to determine what the right collection of words its response will be.
Using an overly simplified analogy, it acts like a dictionary and thesaurus together to determine what you want to extract from its existing dataset. The size of that dataset is where the real merit lies, as almost anything online could be accessed.
And therein lies the problem. The sheer breadth of information available can mean that you don’t always know the validity of the source material.
One of the real areas of concern around GenAI is therefore the lack of visibility over its decision-making process. With no watermarks or breadcrumb trails, it is hard to know how or where the GenAI’s interpretation and deduction has come from.
Yes, it can provide hyperlinks to articles, but its ‘thought process’ is not always clear. If you ask an AI tool about this, it freely admits its own limitations.
The unknown nature of a GenAI tool’s computations can further lead to the concept of ‘hallucination’, i.e. where the tool simply starts to make things up.
A now infamous example of this was seen earlier this year when a lawyer from New York cited case law that simply didn’t exist – only for it to be revealed that the legal research was performed using GenAI rather than standard legal resources. We saw this just last week over here in a ruling from the First-tier Tribunal.
Limiting the dataset can help reduce the risk of this type of hallucination from happening. However, even by being as prescriptive as including your own documents, issues can still arise.
How does it perform in practice?
I recently trialled a GenAI solution by inserting an anonymous, dummy non-disclosure agreement (NDA) into the prompt section and asking it for a summary of the key points. The tool duly produced a paragraph containing bullet points of the key areas from the document. So far, so good.
I then asked it which areas would need negotiating, without saying which party to the agreement I was hypothetically representing. Another set of responses were produced.
When I then suggested that I was the disclosing party, it changed its answers. When I then asked which parts the receiving party would want to negotiate, it changed its answers. At this point however, the responses started to raise alarm bells.
Whilst I was in the middle of this ‘context stuffing’ exercise – a recognised way of trying to get more accurate responses out of a GenAI tool – I noticed that that the responses were becoming shorter, and potentially more misleading.
For example, the template NDA contained the term ‘Affiliate’ (with a capital A) but did not contain a definitions section (due to lack of space to upload the document). The GenAI tool ascribed a definition to that term during its responses. Whilst the attempted definition made sense in everyday language, contract lawyers would ordinarily pounce on the lack of definition in the document and would give that term a specific definition – which could be integral to what you’re trying to achieve with your NDA.
In another test, I inserted the hyperlink to a new piece of legislation and asked a GenAI tool to produce a summary of the new regulations. Within moments a bullet-pointed list of the key provisions was produced.
The list, however, whilst largely following the contents list of the legislation, missed out certain sections. What criteria was it applying to determine which clauses it presented? There is no way of knowing.
From my perspective, they were important omissions. Asking the tool only offered more explanation behind the provisions already shared.
Rather than being a quick fix, the task then changed from being a trial of GenAI’s ability to produce answers to being a verification and corroboration exercise.
Whilst a summary note was produced initially, checking the accuracy of every point became a large task unto itself – to the point that the amount of time spent quantifying the responses could have been better used reading the materials and drafting the summary myself.
A critical balance therefore needs to be made between the time saved by using GenAI versus the time needed to verify and corroborate its outputs. The larger the output, the more room for error.
With the rate that GenAI solutions have been advancing, these issues may eventually fall away, but what these examples highlight right now is an inherent risk with what it deems to be fact, how it presents its interpretation of those facts, and how it came to its conclusions.
How secure is it?
The security of GenAI has been called into question so far, with confidentiality concerns and the potential for data scraping being front and centre.
Certain in-roads are being made on this front as the National Cyber Security Centre (part of GCHQ) has recently published a series of guidelines, in collaboration with multiple international partners, highlighting the “novel security vulnerabilities” and risks that need to be addressed in order to successfully develop, deploy, and operate a secure AI tool.
How does it sit with legal services regulation?
Given the mass of data available to an AI tool with the volume of sites, publications, opinions, articles, blogs, cases, forums etc, it presents a regulatory challenge for lawyers. However, if you limit the dataset itself, are you limiting the capabilities of the tool itself?
The message so far must be: Users Beware
Microsoft Bing Chat’s integration with ChatGPT (now called Copilot) caveats that it should not be used as legal advice. Whilst it can give you different ‘conversation styles’ or ‘voices’ for its output, can your own prompts and its knowledge bank fully encompass the legal industry’s relevant codes of conduct as part of its answers? Maybe.
The prompts and parameters you apply will constantly vary and require you to understand them to operate the tools appropriately. With the technology being so smart but often opaque, users need to be just as savvy to be able to operate it properly.
The legal frameworks within which lawyers operate and conduct themselves require clear thinking and gauging human interactions to be able to work properly. The lack of definitive audit trails as to how GenAI has arrived at its conclusions and/or the inability to assess what the user really wants to achieve does not immediately lend itself to the legal way of working, and without that we will not be able to fully trust in its outputs.
And finally, can it be trusted?
It takes time to build trust. Only with time and experimentation will GenAI be able to produce the right results. Unfortunately, as with any new tech, many fingers may be burnt along the way.
For now – and the foreseeable future – GenAI can only become truly intelligent when applied with human intelligence, due consideration and clear thinking behind it.
This article is not to decry the merits and incredible advances of AI – moreover, it is a cautionary tale. The development of AI has been described by many as being as important as the discovery of electricity. Let’s just make sure we don’t get a nasty shock along the way.